Artificial intelligence has achieved remarkable feats, from mastering chess to predicting protein folding and even distinguishing between cats and dogs. Yet, it's the captivating realm of generative AI that's currently capturing global imagination.
In January, ChatGPT made history by becoming the fastest-growing software program ever, amassing a staggering hundred million users in less than two months since its public debut. This surge gave rise to a flurry of competitors, including proprietary contenders like Google's Bard platform and open-source alternatives like Berkeley's Koala. Notably, this fervor has initiated an arms race among tech giants Microsoft and Google, along with a substantial boost to the AI chip industry, led by Nvidia.
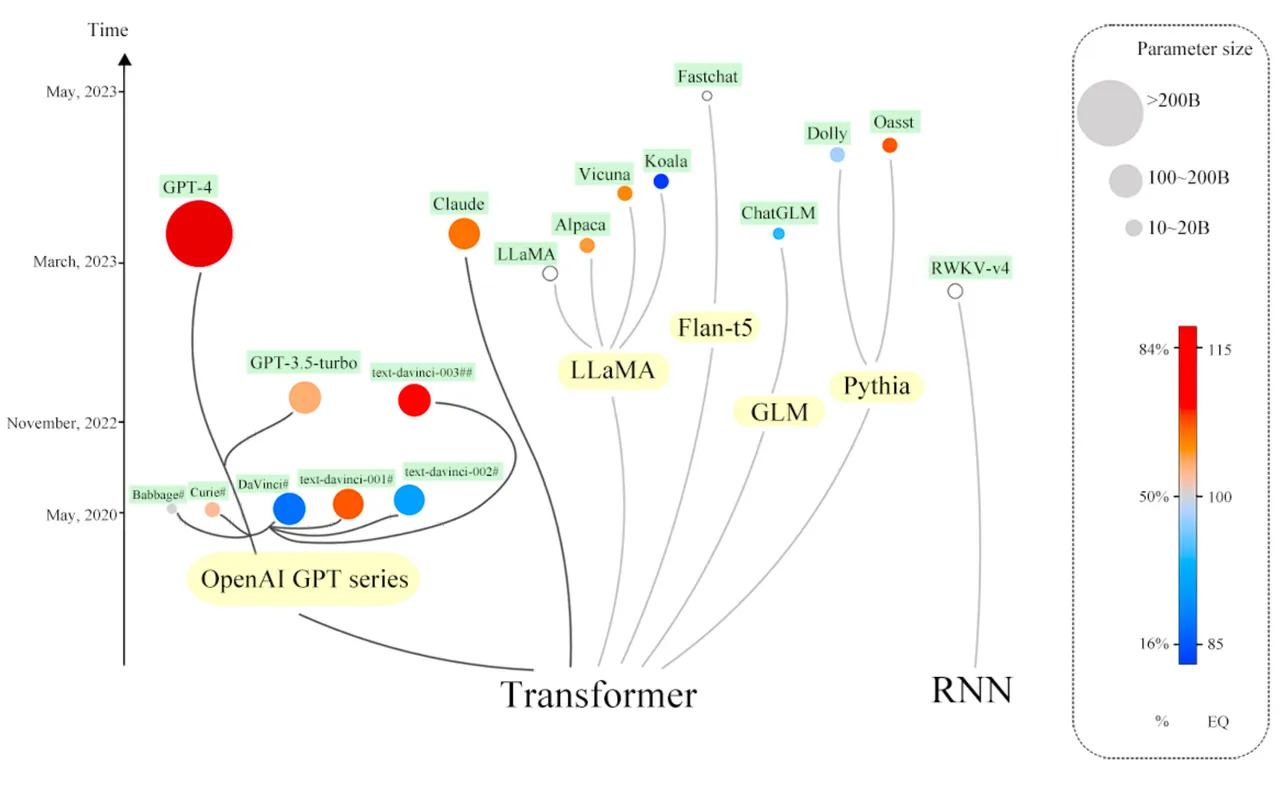
The magnetic appeal of generative AI lies in its capacity to do more than assign numeric scores like its predecessors. Instead, it replicates facets of the real world, producing paragraphs, images, and even the architecture of computer programs, thereby reflecting society's creations.
The evolutionary trajectory of generative AI is set to skyrocket. Today's generative programs will appear rudimentary compared to the multifaceted capabilities expected by the year's end, diversifying their output across various modalities.
The Rise of Multi-Modal Generative AI
The next frontier in computer science is embracing mixed modalities, where AI seamlessly combines text, images, 3D spatial data, sounds, videos, and entire computer functionalities. This transition promises more powerful applications and advances the concept of continuous learning, potentially propelling robotics into new realms.
Naveen Rao, the founder of AI startup MosaicML, notes that ChatGPT while entertaining, is essentially a demonstration. The next phase involves moving beyond personal "Copilots" to collaborative tools that enhance teamwork and storytelling, transcending solitary usage.
One critical aspect currently missing is multi-modality in AI models, as they primarily interpret the world through text alone. Modalities refer to the nature of input and output, whether text, image, or video, and the future hinges on integrating various modalities effectively.
Jim Keller, CEO of AI chip startup Tenstorrent, believes that handling mixed modalities will become a pivotal demand in AI development.
Bridging the Modal Gap
Large language models at the core of AI technology transform text into tokens, quantified mathematical representations. Similarly, image generation employs processes like Stable Diffusion to corrupt images with noise and then reconstruct the original image, training neural networks to produce high-fidelity images.
These processes of recovering missing or corrupted data extend to multiple modalities. For instance, University of Washington biologists used RFdiffusion to corrupt protein amino acid sequences, enabling neural networks to generate synthetic proteins with desired properties. This approach accelerates the development of novel antibodies for diseases.
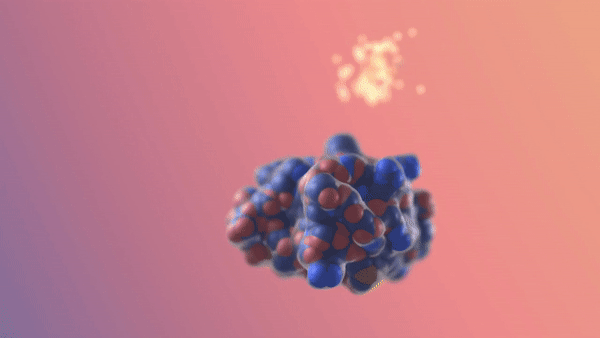
Multi-Modal Innovation
Companies like Stability AI and OpenAI are pioneers in exploring various modalities. Stability AI, for instance, has labs dedicated to audio, code generation, and even biology. The magic happens when these modalities intersect. Researchers found that combining text-based prompts with image refinement improved image quality dramatically.
Other AI researchers, such as those at Meta, propose combining text and image models to perform tasks that involve both, like identifying objects in images or generating captions.
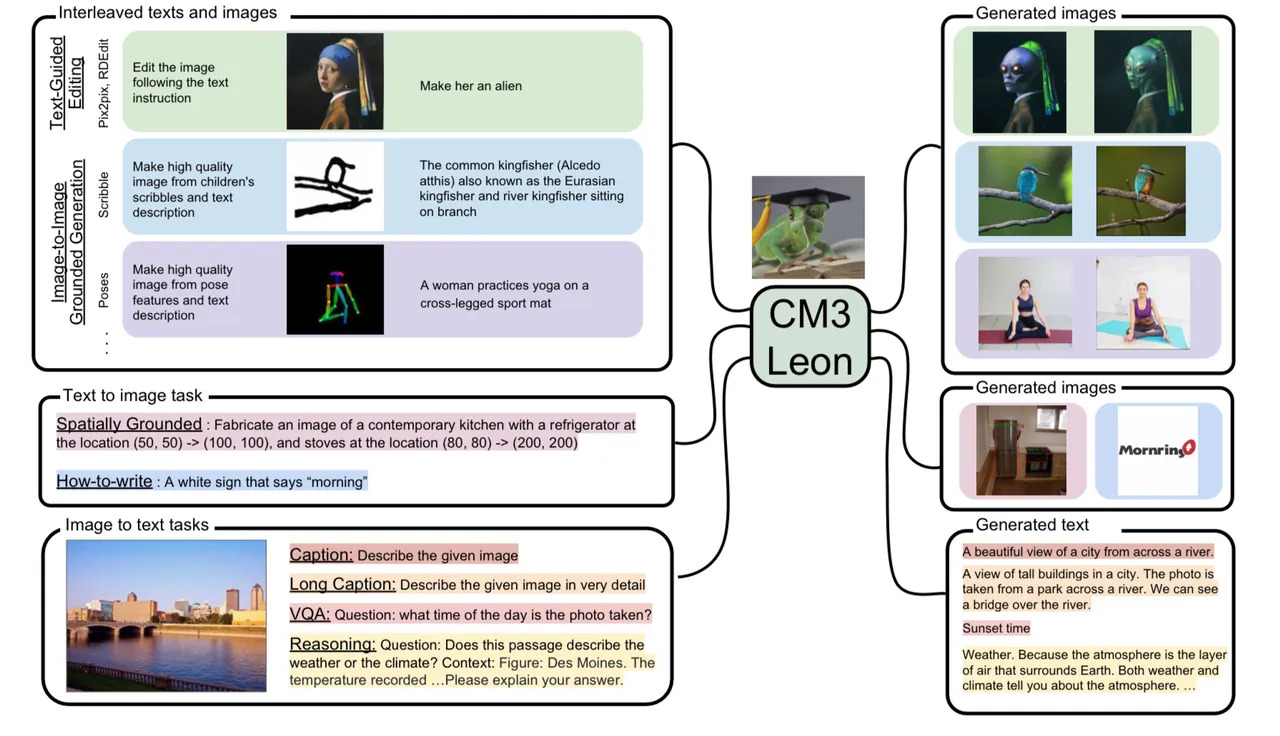
Expanding the Worldview
Integrating multiple modalities enriches neural networks' understanding of the world. This fusion of information could enable AI systems to mimic the human concept of "stereognosis," allowing them to understand the world through multiple senses, leading to richer, more sophisticated reasoning abilities.
Carnegie Mellon University's "High-Modality Multimodal Transformer" combines text, image, video, speech, database table information, and time series data, demonstrating performance improvements with each added modality.
The Meta-Transformer developed at the Chinese University of Hong Kong goes even further, incorporating a dozen modalities, including 3D vision and hyperspectral sensing data.
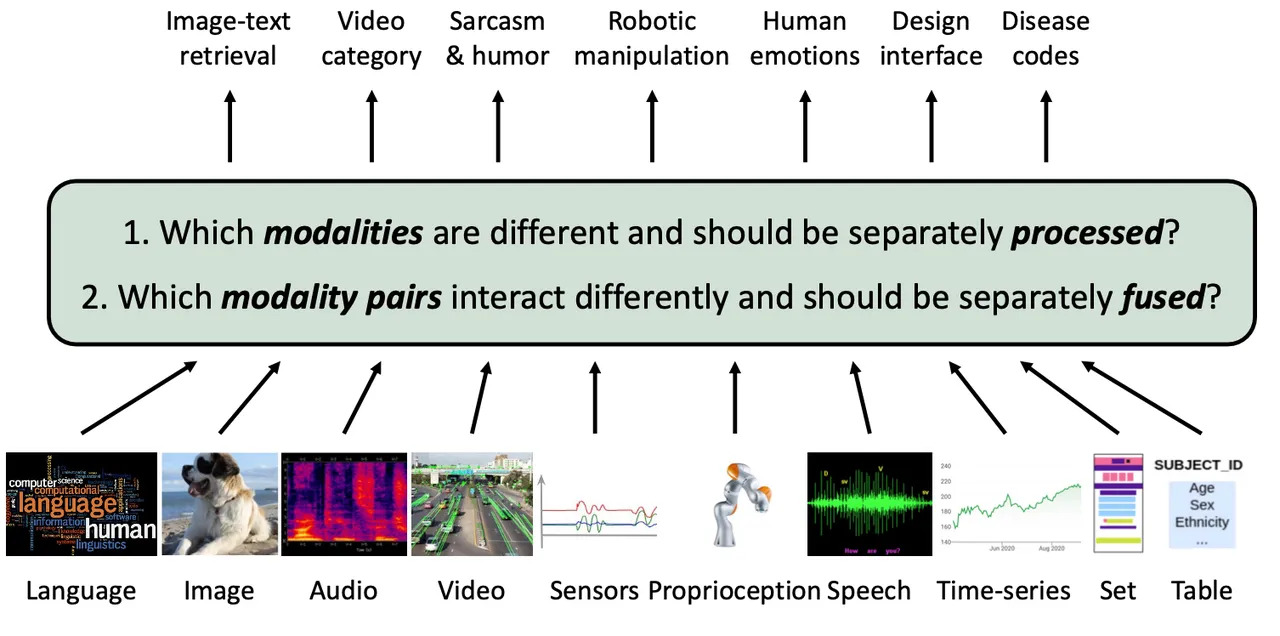
Multi-Modality Applications
Multi-modality's immediate impact will be seen in enhancing outputs beyond the demo stage, particularly in domains like storytelling. By combining language and image attributes, AI systems can generate images that align with text prompts, facilitating the creation of dynamic children's storybooks.
Furthermore, AI can create storybooks where characters traverse different scenarios image by image, introducing the concept of "Iterative Coherent Identity Injection."
Beyond Storybooks: Robotic Advancements
The convergence of modalities holds significant promise for embodied AI, particularly in robotics. Multi-modal neural networks can already generate high-level robot commands, bridging the gap between human instructions and robotic actions.
Projects like Google's PaLM-E demonstrate how AI models can break down complex instructions into atomic actions, enabling robots to understand and execute tasks efficiently.
Continuous Learning and Memory Expansion
As multi-modality extends to video, audio, point clouds, and more, it challenges the traditional distinction between training and inference in AI models. Generative models are evolving to seamlessly integrate the two processes, allowing for continuous learning.
Expanding data sources to thousands of images and hours of video will require AI models to develop explicit memory capabilities, a departure from the current "short-term" memory. This expanded context will lead to more tailored and personalized responses.
The Future of Generative AI
As generative AI matures, it's poised to unlock a world of possibilities. From collaborative multi-modal models to advancements in robotics, the fusion of modalities is set to reshape the landscape of technology and artificial intelligence, ushering in an era of continuous learning and enriched human-machine interactions.