Etherscan, the Ethereum block explorer and analytics platform, has unveiled a cutting-edge tool named "Code Reader" that leverages the power of artificial intelligence (AI) to retrieve and analyze the source code of specific contract addresses. Developed in collaboration with OpenAI's large language model, Code Reader offers users valuable insights into the underlying contract's source code files.
By inputting a prompt, users can generate a response from Code Reader, providing them with a comprehensive understanding of the contract's code. The tool's tutorial page emphasizes that a valid OpenAI API Key and sufficient usage limits are necessary to utilize Code Reader, assuring users that their API keys are not stored by the tool.
The application of Code Reader spans various use cases, including gaining deeper insights into contract code through AI-generated explanations, obtaining comprehensive lists of smart contract functions related to Ethereum data, and comprehending the contract's interaction with decentralized applications. Users are also able to read through specific source code files and modify them directly within the user interface (UI) before sharing them with the AI.
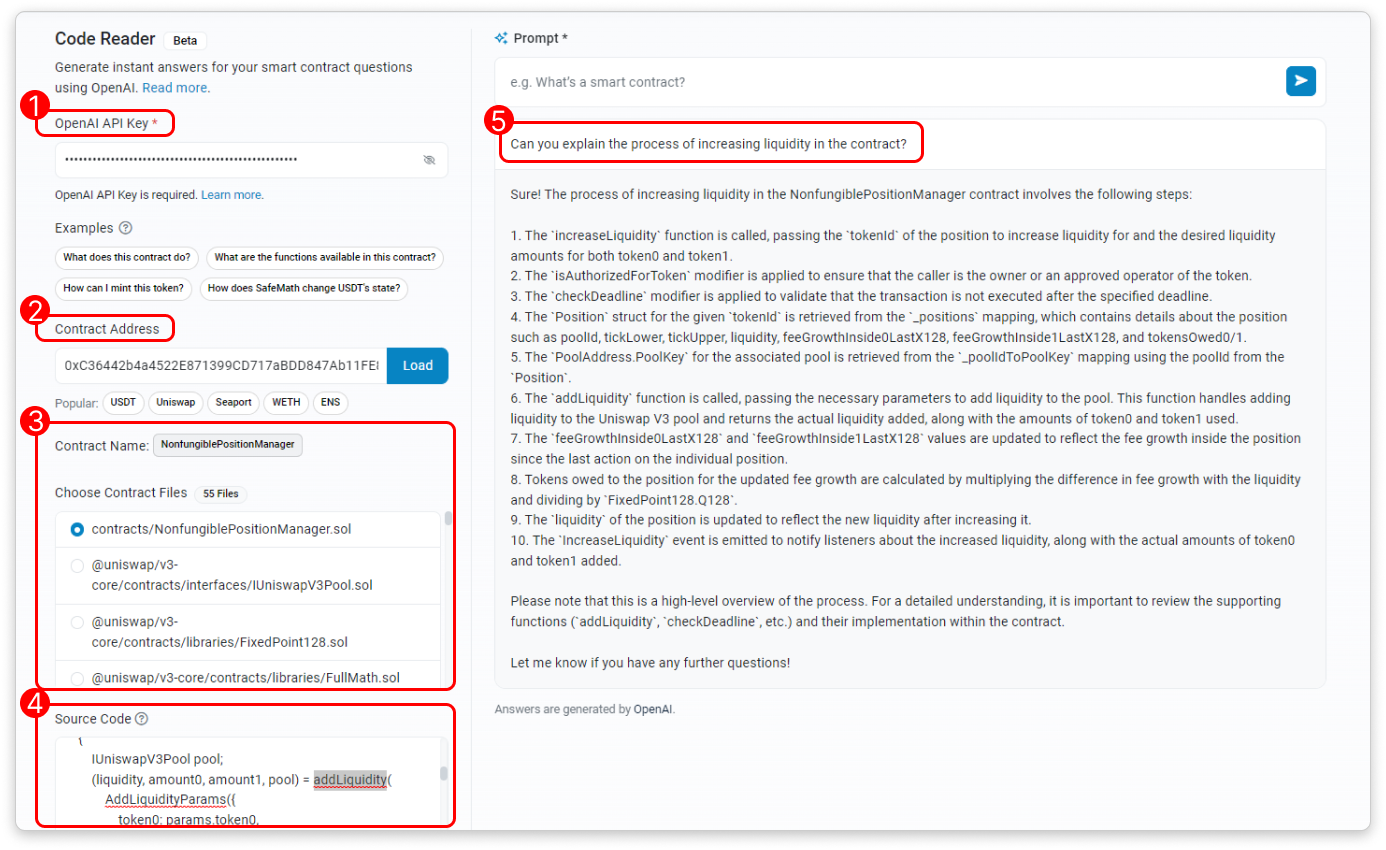
Despite the flourishing AI landscape, experts caution about the practicality of current AI models. Foresight Ventures, a Singaporean venture capital firm, recently published a report stating that computing power resources will be a significant battleground in the next decade. As demand for training large AI models in decentralized distributed computing power networks grows, researchers have identified several constraints, including complex data synchronization, network optimization, and concerns regarding data privacy and security.
To illustrate these challenges, Foresight researchers provided an example involving the training of a massive model consisting of 175 billion parameters using single-precision floating-point representation. The process would require approximately 700 gigabytes of data. However, in distributed training, these parameters need to be frequently transmitted and updated between computing nodes. For instance, if there are 100 computing nodes and each node has to update all parameters at each unit step, the model would necessitate the transmission of 70 terabytes of data per second. This amount surpasses the capacity of most networks.
In light of these findings, the researchers conclude that, in most scenarios, smaller AI models remain a more practical choice. They caution against prematurely overlooking smaller models amidst the fear of missing out (FOMO) on large models.